|
I'm facing a problem with an SSIS package.
- A query is executed to obtain some data from the DataBase (SQL Server 2008) (Data Flow Task executed)
- Export the data extracted to an Excel 97-2003 spreadsheet (.xls) using Excel Destination
As most of you know the xls files are limited per sheet to 65,536 rows by 256 columns. So when the query extracts more than the records limit (65,536), the Excel Destination Step fails.
The Solution is
Here is one possible option that you can use to create Excel worksheets dynamically using the SSIS based on how many number of records you want to write per Excel sheet. This doesn't involve Script tasks. Following example describes how this can be achieved using Execute SQL Tasks, For Loop container and Data Flow Task. The example was created using SSIS 2008 R2 .
Step-by-step process:
In SQL Server database, run the scripts provided under SQL Scripts section. These scripts will create a table named dbo.SQLData and then will populate the table with multiplication data from 1 x 1 through 20 x 40 , thereby creating 800 records. The script also creates a stored procedure named dbo.FetchData which will be used in the SSIS package.
On the SSIS package, create 9 variables as shown in screenshot #1. Following steps describe how each of these variables are configured.
Set the variable ExcelSheetMaxRows with value 80. This variable represents the number of rows to write per Excel sheet. You can set it to value of your choice. In your case, this would be 65,535 (you might want to leave 1 row for header column names).
Set the variable SQLFetchTotalRows with value SELECT COUNT(Id) AS TotalRows FROM dbo.SQLData . This variable contains the query to fetch the total row count from the table.
Select the variable StartIndex and choose Properties by pressing F4. Set the propertyEvaluateAsExpression to True and the property Expression to the value (@[User::Loop] * @[User::ExcelSheetMaxRows]) + 1 . Refer screenshot #2.
Select the variable EndIndex and choose Properties by pressing F4. Set the propertyEvaluateAsExpression to True and the property Expression to the value (@[User::Loop] + 1) * @[User::ExcelSheetMaxRows] . Refer screenshot #3.
Select the variable ExcelSheetName and choose Properties by pressing F4. Set the property EvaluateAsExpression to True and the property Expression to the value "Sheet" + (DT_WSTR,12) (@[User::Loop] + 1) . Refer screenshot #4.
Select the variable SQLFetchData and choose Properties by pressing F4. Set the propertyEvaluateAsExpression to True and the property Expression to the value "EXEC dbo.FetchData " + (DT_WSTR, 15) @[User::StartIndex] + "," + (DT_WSTR, 15) @[User::EndIndex] . Refer screenshot #5.
Select the variable ExcelTable and choose Properties by pressing F4. Set the propertyEvaluateAsExpression to True and the property Expression to the value provided underExcelTable Variable Value section. Refer screenshot #6.
On the SSIS package's Control Flow tab, place an Execute SQL Task and configure it as shown in screenshots #7 and #8. This task will fetch the record count.
On the SSIS package's Control Flow tab, place a For Loop Container and configure it as shown in screenshot #9. Please note this is For Loop and not Foreach Loop. This loop will execute based on the number of records to display in each Excel sheet in conjunction with the total number of records found in the table.
Create an Excel spreadsheet of Excel 97-2003 format containing .xls extension as shown in screenshot #10. I created the file in *C:\temp*
On the SSIS package's connection manager, create an OLE DB connection named SQLServer pointing to SQL Server and an Excel connection named Excel pointing to the newly created Excel file.
Click on the Excel connection and select Properties. Changes the property DelayValidation from False to True so that when we switch to using variable for sheet creation in Data Flow Task, we won't get any error messages. Refer screenshot #11.
Inside the For Loop container, place an Execute SQL Task and configure it as shown in screenshot #12. This task will create Excel worksheets based on the requirements.
Inside the For Loop container, place a Data flow task. Once the tasks are configured, the Control Flow tab should look like as shown in screenshot #13.
Inside the Data Flow Task, place an OLE DB Source to read data from SQL Server using the stored procedure. Configure the OLE DB Source as shown in screenshots #14 and #15.
Inside the Data Flow Task, place an Excel Destination to insert the data into the Excel sheets. Configure the Excel destination as shown in screenshots #16 and #17.
Once the Data Flow Task is configured, it should look like as shown in screenshot #18.
Delete the Excel file that was created in step 12 because the package will automatically create the file when executed. If not deleted, the package will throw the exception that Sheet1 already exists. This example uses the path C:\temp\ and screenshot #19 shows there are no files in that path.
Screenshots #20 and #21 show the package execution inside Control Flow and Data Flow tasks.
Screenshot #22 shows that file ExcelData.xls has been created in the path C:\temp. Remember, earlier this path was empty. Since we had 800 rows in the table and we set the package variable ExcelSheetMaxRows to create 80 rows per sheet. Hence, the Excel file has 10 sheets. Refer screenshot #23.
NOTE: One thing that I haven't done in this example is to check if the file ExcelData.xls already exists in the path C:\temp. If it exists, then the file should be deleted before executing the tasks. This can be achieved by creating a variable that holds the Excel file path and use a File System Task to delete the file before the first Execute SQL Task is executed.
ExcelTable Variable Value:
"CREATE TABLE `" + @[User::ExcelSheetName] + "`(`Id` Long, `Number1` Long, `Number2` Long, `Value` Long)"
SQL Scripts:
--Create table
CREATE TABLE [dbo].[SQLData](
[Id] [int] IDENTITY(1,1) NOT NULL,
[Number1] [int] NOT NULL,
[Number2] [int] NOT NULL,
[Value] [int] NOT NULL,
CONSTRAINT [PK_Multiplication] PRIMARY KEY CLUSTERED ([Id] ASC)) ON [PRIMARY]
GO
--Populate table with data
SET NOCOUNT ON
DECLARE @OuterLoop INT
DECLARE @InnerLoop INT
SELECT @OuterLoop = 1
WHILE @OuterLoop <= 20 BEGIN
SELECT @InnerLoop = 1
WHILE @InnerLoop <= 40 BEGIN
INSERT INTO dbo.SQLData (Number1, Number2, Value)
VALUES (@OuterLoop, @InnerLoop, @OuterLoop * @InnerLoop)
SET @InnerLoop = @InnerLoop + 1
END
SET @OuterLoop = @OuterLoop + 1
END
SET NOCOUNT OFF
--Create stored procedure
CREATE PROCEDURE [dbo].[FetchData]
(
@StartIndex INT
, @EndIndex INT
)
AS
BEGIN
SELECT Id
, Number1
, Number2
, Value
FROM (
SELECT RANK() OVER(ORDER BY Id) AS RowNumber
, Id
, Number1
, Number2
, Value
FROM dbo.SQLData
) T1
WHERE RowNumber BETWEEN @StartIndex AND @EndIndex
END
GO
Screenshot #1:
Screenshot #2:
Screenshot #3:
Screenshot #4:
Screenshot #5:
Screenshot #6:
Screenshot #7:
Screenshot #8:
Screenshot #9:
Screenshot #10:
Screenshot #11:
Screenshot #12:
Screenshot #13:
Screenshot #14:
Screenshot #15:
Screenshot #16:
Screenshot #17:
Screenshot #18:
Screenshot #19:
Final Package is look like this
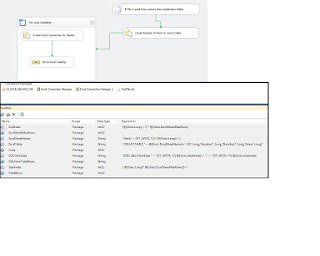
SSIS - Merge join vs Lookup transform Performance – Case Study
Hi ,
In this post i'll compare the two join components: the merge join and the lookup join with the relation join in order to determine which solutions is the best possible way to solve your problem regarding joining data from certain data sources. This post is based on SQL Server 2008 R2.
Merge Join The merge join is used for joining data from two or more datasources. The more common ETL scenarios will require you to access two or more disparate datasources simultaneously and merge their results together into a single datasource. An example could be a normalized source system and you want to merge the normalized tables into a denormalized table. The merge join in SSIS allows you to perform an inner or outer join in a streaming fashion. This component behaves in a synchronous way. The component accepts two sorted input streams, and outputs a single stream, that combines the chosen columns into a single structure. It’s not possible to configure a separate non-matched output.
Lookup The lookup component in SQL Server Integration Services allows you to perform the equivalent of relational inner and outer hash joins. It’s not using the algorithms stored in the database engine. You would use this component within the context of an integration process, such as a ETL Layer that populates a datawarehouse from multiple non equal source systems. The transform is written to behave in a synchronous manner in that it does not block the pipeline while it’s doing its work, mostly. In certain cache modes the component will initially block the package’s execution for a period of time.
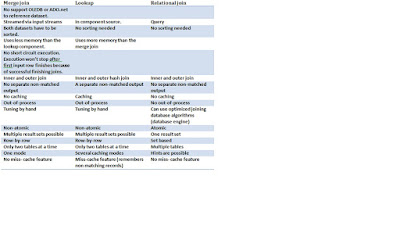
Conclusion: There's only one reason for using the merge join and that is a lack of memory, otherwise always use the lookuptransform. There's done lot of improvement of the lookup transform in SQL Server 2008.
Case Study on Merge Join Vs Lookup Performance - I:
TheViewMaster wrote:
|
So here we go: I'm running the tests on my workstation WinXP, 2.93GHz, 2.5gb ram. The DB is accessed over the LAN. Test1 (Lookup): Source: multi-flat-file source (4 .txt's) with total of 175513 records and 88 columns Lookup is a query access table 248250 records pulling 61280 records and about 25 columns 2 outputs - Listing Found (56523 rows) and Error Listing Not found (118990 rows) Also lookup is Full Cache mode and gives Warning: found duplicate key values. Result: Finished, 4:11:00 PM, Elapsed time: 00:00:15.437 Note: Memory usage of PC peaked at 1.8GB with CPU usage jumping to 100% once.
Test 2 (Merge Join): 1st Source: multi-flat-file source (4 .txt's) with total of 175513 records and 88 columns 2nd source: OLE DB Source with query access table 248250 records pulling 61280 records and about 25 columns with ORDER BY ID. Out put is marked sorted by ID column. 1st source is Sorted using "Sort transform". Then "Merge Joined" with ole db via Left outer join (Sort on left) Then "Conditional Split" based on ISNULL(oledbsource.ID) Result: Finished, 4:49:33 PM, Elapsed time: 00:01:14.235 Note: Memory usage of PC peaked at 2.6GB with CPU usage jumping to 100% twice.
Test3 (Script Transform) - Source: multi-flat-file source (4 .txt's) with total of 175513 records and 88 columns Script transform to do a lookup based on key column for each row in pipeline. Result: Cancelled after 30 minutes of processing - during which it had process 11547 records (out of 175513) Note: Memory usage was stable around 1GB and CPU near 5% usage
My Conclusion: Although I was concerned with the performace of lookup transform - for testing whether data to be inserted or updated - it seems thats not the culprit - the root of evil seems to be OLE DB update command and OLE DB Destination source (atm we r using SQL 2000 db - upgrading to 2005 soon). Although Script transform consumed least amount of machine resources - executing 100K+ sql queries against db will take too long. Although merge join Elapse time is not bad - resource usage and 3 more steps than lookup are negatives. So i think next weekends performance testing is how to make faster INSERTs/UPDATEs to DB
|
|
|
Check it once through MSBI Online Training Hyderabad for more information.
ReplyDeleteTo split a large or huge dataset into multiple Excel spreadsheets using an SSIS (SQL Server Integration Services) package, you can follow a few steps. First, create a data flow task in the SSIS package and configure the source component to retrieve the dataset you want to split. Next, Best travel cameras, add a conditional split transformation that allows you to define rules for splitting the data based on specific criteria, such as a column value or row count. Then, create multiple Excel destination components, each corresponding to a specific split condition, and configure them to write the data to separate Excel spreadsheets. Finally, connect the conditional split transformation to the respective Excel destinations, ensuring that each split portion of the dataset is directed to the appropriate spreadsheet. This approach allows you to efficiently divide a large dataset into multiple manageable Excel files using the capabilities of SSIS.
ReplyDeletecheap camera for photography